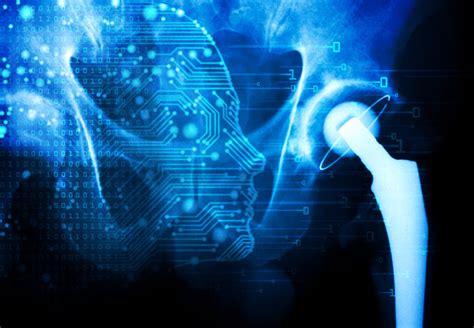
Artificial Intelligence in the Musculoskeletal Space (presented by Ingrid Vasiliu-Feltes
CEO Softhread) published by the Journal of Orthopaedic Experience & Innovation
This lecture was from the Orthopaedic Value Based care Conference 2021.

This article will address the use of artificial intelligence (AI) in the musculoskeletal of space.

The overview is as follows. I will start with defining AI, and sharing opportunities and challenges we have experienced in the AI landscape. Following this, I will cover the potential impact of AI, provide a 360° view and focus in on the specific AI applications in the musculoskeletal space. We will specifically look at some pain points and how AI can augment the value proposition. Last, I will focus on some future directions that would benefit from collaborative efforts.

Before we start, I always like to define AI. It is often used by many in social media or even in scientific publications in a way that is causing confusion. Whenever we mention the term AI, it is essential to determine if are we talking about narrow AI, general AI, artificial general intelligence, or artificial superintelligence? All are different in terms of applications, ethical concerns, value propositions, technological deployments, and they can all be used with the portfolio of tools we have available.

Unfortunately, many publications have not always highlighted the nuances and complexities of the AI portfolio of tools and methods. The following is a summary of the key tools and methods that we need to be aware of when discussing healthcare and the life sciences.
Symbolic AI, rules-based AI, robotics, computer sensing, knowledge engineering, natural language processing, and machine learning are some of the major categories that can be deployed in healthcare and the life sciences.

Machine learning is another term that has caused a lot of confusion and unfortunately, a lot of distrust in the industry. So again—it is very important to differentiate the types of machine learning. Depending on the situation, the primary ones that can be helpful in healthcare or the life sciences are deep learning, reinforcement learning, neural networks, and the ones that always cause a lot of attention and a lot of discussions— general adversarial networks.
Each of the above types of learning and tools are terrific, but we want to delineate the type of case in which we use a specific learning type/tool. In publications where it is said that one method is superior than another, we should ensure that the learning type/tool is highlighted. The learning type/tool always depends on the specifications and what type of case you want to deploy them with—one method is no better than the other. All of them can be great—as long as you select the right tool and the right method for the use case that you have in mind.

The types of learning include supervised and unsupervised learning, with the former having different inputs and desired outputs labeled versus in unsupervised learning where you have no labeled outputs. In supervised learning, regression and clustering are the most common approaches. In unsupervised learning, exploratory analysis and dimensionality reduction are very common major categories.

Now we will address the opportunities for AI in healthcare and particularly in the musculoskeletal space. It is helpful to categorize AI opportunities into non-clinical and clinical. In the non-clinical section of healthcare, there is the optimization of healthcare information system processes, the optimization of healthcare management processes, as well as the streamlining the healthcare payment process.
When we talk about clinical applications of AI, the opportunities are even broader. The most publicized uses of AI to date have been in screening, diagnosis and treatment, recovery, image segmentation, and image resolution.

Of all the challenges that we are encountering in the AI space, trust is the number one issue. This is because of widespread myths, as well as a lack of proper education at various stages during our medical or professional education. I think there is a tremendous digital literacy lag and digital fluency lag that we see throughout.
In addition, compliance and legal hurdles relating to AI have been accentuated. Those are always hurdles in anything we do in healthcare and the life sciences, but when it involves deploying AI in healthcare, there is another layer of complexity.
Last but not least, cost and the difficulty of tracking the return on investment (ROI) from AI is a major element. Often, enterprises and healthcare institutions or any other life sciences organizations deploy AI as a pilot effort, which seems to alleviate some of the concerns about risk. However, this means that you cannot track ROI from your project because you just have little pilots here and there and you can never have a comprehensive assessment of what long-term benefits you can reap from the AI deployment.
Unfortunately, technological advancements severely outpace regulatory guidelines. While this has been the case for some time, with the rapid acceleration of emerging technologies being adopted in all industries, it has become a very significant trend.

Now we turn to the 360° opportunities of deploying AI in the musculoskeletal space. AI can be deployed in healthcare management, clinical care, and in the imaging value chain, the latter of which has received the most attention in the published literature. As for the value chain, it would be segregated into interpretive or non-interpretive, both of which can be very impactful and valuable when you deploy AI.

In using AI, we must try to address various pain points as opposed to just deploying AI because it is the hot new thing in healthcare. We should have crystal clear pain points so that we can select the right tools and the right specifications (like we mentioned for the technical inputs and outputs). Some of the major pain points that provide opportunities for improvement are image pre-processing, image acquisition, image analysis, documentation and report generation, consistency, sensitivity, specificity, and validity. Each one of these is highly amenable to AI intervention.

When we discuss opportunities in the healthcare management space for musculoskeletal care, we can address credentialing, certification, performance improvement for providers, clinical care, personnel, payments, quality metrics, and value-based metrics. Although this is not something that frequently appears in the literature, there are many companies that are deploying AI on the back end and you just are not aware of it. It has become quite a big trend over the last two years to embed AI in some of these healthcare management processes. Thus, while the majority of our publications focus on the clinical element, it is important to pay attention to this element as well.

In the noninterpretive space, the impact can be seen when AI is deployed as virtual assistants for providers and for clinical care. This involves test ordering, automated exam protocols, optimized scheduling, optimized case prioritization, dynamic case matching, shorter acquisition times, reduced artifact dosing, report generation customization, and automation. Throughout the industry, there are numerous examples of startups successfully deploying AI.

Regarding the interpretative impact, there are many published examples where AI has been used for bone age determination, body composition measurements, osteoporosis screening, fracture identification, the evaluation of segmental spine pathology, detection of metastasis, the diagnosis of tumors of primary bone or soft tissue, and the grading of osteoarthritis.
Unfortunately, all of these have been done only in small studies or small pilots or they are still in progress. Thus, our goal is to learn from these and then collaborate on the deployment of AI as a larger-scale project. Using this approach would help avoid some of the previously mentioned downfalls such as inconsistency, lack of ability to track ROI, and so forth.

As for AI’s value proposition, when you try to measure it or explain it to the c-suite and convince someone them to use AI, it is important to structure the value proposition analysis. The report should be based on preclinical clinical and post-clinical because the cost centers and budgets are very different for each of these sections as viewed from the patient journey perspective.
Thus it is essential to highlight the value proposition, not only the soft but also the dollar side of it based on these domains. For the preclinical side, it is fairly obvious that works optimization is very helpful. On the clinical side, I just highlighted two segments that receive a lot of attention in most publications—care pathway optimization and improved clinical decision support. This is why I often propose that in healthcare we should change from “artificial intelligence” to “augmented” as the former term can have negative connotations.
If we use “augmented intelligence,” it implies that the human element will always be there. We need physician expertise and clinical expertise of all our colleagues that are involved in the 360° healthcare industry. But it is essential to understand that it would be an augmentation of our current expertise and our current efforts. Augmented care will not completely replace the human element—at least in this era. The use of such care in the post-clinical phase is also important as we must demonstrate improved quality outcomes and then improved long-term ROI.

When conducting a feasibility analysis and therefore the value proposition, it is helpful to show it by technology deployed, particularly for the musculoskeletal space. The value proposition for deploying AI in the X-ray space is different than that of MRI or CT scans. And again, that is crucial when you design a strategic roadmap and implementation.

When considering the value proposition by domain and discussing this with the c-suite of hospitals, it is helpful to segregate it by imaging value proposition, surgical impact, perioperative care impact, foster care, the related general clinical care domains, as well as Healthcare Information Management Systems. Each of those has different decision stakeholders and different cost centers. While it does add a bit of work, it can yield great results.

I would like to highlight a very clear trend that I have observed over the last 15 to 18 months: most projects that I see in startups or enterprise AI involve converging technologies. You very rarely see just AI deployed as a standalone technology. There is some vague combination of artificial intelligence plus augmented reality, virtual reality, mixed reality, and lately, extended reality. This ranges from haptic gloves that can measure if a surgeon makes the right movements as per best practices for many other interactive surgical procedures where you can use any of these technologies in combination. There are several companies worldwide that have made huge strides in designing the OR of the future.
3D printing, which was all the rage several years ago and then experienced a lull, is experiencing a resurgence in adoption. I have seen many pilot programs that deploy AI and 3D printing to optimize care. Nanotech, holograms, genomics—we will see all of these increasing over the next few years. We might have to rethink how we deploy these technologies because there is a melding and tremendous convergence underway. This is critical because our areas of expertise—and healthcare in general—will also need to adjust the way we deploy these approaches.
